How Quantum Neural Networks Are Shaping the Future of AI | Neuroba
- Neuroba
- Jan 1
- 5 min read
Artificial Intelligence (AI) has seen rapid advancements over the past few decades, with machine learning, deep learning, and neural networks driving much of the progress. However, despite their success, traditional computational models still face limitations in terms of scalability, processing speed, and efficiency. Enter Quantum Neural Networks (QNNs)—a transformative concept that combines the principles of quantum computing with neural networks to revolutionize the future of AI. This blog explores how Quantum Neural Networks are shaping the future of AI, providing new frontiers for computational capabilities, and how Neuroba is actively involved in pioneering this integration.
Understanding the Basics of Quantum Neural Networks
To appreciate how QNNs are reshaping AI, it’s important to first understand the fundamental principles of quantum computing and neural networks.
Quantum Computing: Harnessing the Power of Quantum Mechanics
Quantum computing leverages the principles of quantum mechanics, which govern the behavior of particles at microscopic scales. Quantum computers use quantum bits (qubits) instead of traditional bits, allowing them to represent and process information in ways that classical computers cannot. Qubits can exist in multiple states simultaneously due to superposition, and they can be entangled, meaning the state of one qubit can depend on the state of another, even across vast distances.
This unique computational power enables quantum computers to solve specific types of problems much faster than classical computers. While current quantum computers are still in the early stages of development, the potential for quantum computing to revolutionize fields such as cryptography, material science, and machine learning is immense.
Neural Networks: Mimicking the Human Brain
Neural networks are a key component of AI, modeled after the human brain’s structure and function. These networks consist of layers of interconnected nodes (neurons), where each node processes data and passes the output to the next layer. Deep learning, a subset of machine learning, uses multi-layered neural networks to learn complex patterns and features from vast amounts of data.
However, traditional neural networks, despite their effectiveness in tasks like image recognition, natural language processing, and predictive analytics, are still limited by classical computing power. Their computational complexity grows exponentially with the increase in data, making it challenging to scale efficiently.
The Synergy of Quantum and Neural Networks
Quantum Neural Networks combine the unique capabilities of quantum computing with the structure of traditional neural networks. By leveraging quantum properties like superposition and entanglement, QNNs enable the processing of massive datasets and complex computations at speeds far beyond what classical systems can achieve. These networks are particularly well-suited for tasks involving high-dimensional data or optimization problems, which are common in AI.
How Quantum Neural Networks Are Transforming AI
The integration of quantum computing into neural networks opens up new opportunities for AI that were previously unattainable with classical systems. Below are some key areas where Quantum Neural Networks are making a significant impact.
1. Enhancing Computational Efficiency
Traditional neural networks often struggle with computational inefficiency, particularly when dealing with large datasets or complex models. Training deep neural networks can require enormous computational resources, leading to long processing times and high energy consumption.
Quantum Neural Networks, by utilizing quantum parallelism, can process vast amounts of data simultaneously. This allows QNNs to perform tasks such as training on large datasets much more efficiently than classical systems. Quantum parallelism reduces the number of operations needed to solve a problem, accelerating computation and potentially reducing energy consumption, which is critical for sustainability in AI applications.
2. Improving Pattern Recognition and Feature Extraction
In fields such as image recognition, speech processing, and drug discovery, AI systems are tasked with recognizing patterns in complex datasets. Traditional neural networks rely on brute-force processing to detect patterns, which can be time-consuming and computationally expensive.
QNNs, on the other hand, can exploit quantum interference and superposition to explore many possible solutions in parallel. This enables them to recognize patterns more efficiently, even in highly intricate datasets. By processing multiple patterns simultaneously, QNNs can uncover relationships and features that might be overlooked by classical neural networks, significantly improving accuracy in tasks such as classification, clustering, and anomaly detection.
3. Boosting Optimization Algorithms
Optimization is a key component of many AI applications, from training deep learning models to solving complex decision-making problems. Traditional optimization algorithms, such as gradient descent, can be slow and may get stuck in local minima, limiting their effectiveness.
Quantum computing offers powerful optimization techniques such as quantum annealing, which can solve complex optimization problems more efficiently by exploring the solution space in a non-linear way. Quantum Neural Networks, by combining quantum optimization methods with neural network architectures, enable AI systems to find better solutions in less time, overcoming the limitations of classical algorithms.
4. Solving Complex Simulations
In scientific fields such as material science, climate modeling, and drug discovery, AI is often used to simulate and predict outcomes based on complex systems. These simulations require extensive computational resources, especially when dealing with large-scale molecular interactions or high-dimensional datasets.
QNNs have the potential to revolutionize these simulations by leveraging quantum mechanics to simulate quantum systems more efficiently. The ability to model quantum phenomena directly within neural networks opens up new possibilities for simulating complex molecular structures, understanding chemical reactions, and predicting the behavior of quantum materials.
5. Creating Robust AI Models
Traditional AI systems are often vulnerable to adversarial attacks, where small, intentional perturbations to input data can lead to incorrect predictions. Quantum Neural Networks offer the potential for more robust AI systems due to their ability to process information in multiple states simultaneously, making it harder for adversarial manipulations to significantly affect the network’s performance.
Furthermore, the inherent uncertainty in quantum computing may provide a level of noise immunity, leading to more resilient AI models that can perform well even in the presence of errors or incomplete information.
Neuroba’s Role in Quantum Neural Network Development
At Neuroba, we recognize the transformative potential of Quantum Neural Networks in the realm of AI. As pioneers in neurotechnology, we are at the forefront of exploring how quantum computing can enhance the development of intelligent systems that bridge the gap between human cognition and machine learning. Through our ongoing research and collaborations with leading quantum computing institutions, we aim to integrate quantum techniques into our neural networks to enable faster, more efficient, and more intelligent AI solutions.
Our focus is on harnessing the power of quantum computing to push the boundaries of what AI can achieve, creating smarter and more adaptable systems that can learn and evolve in real-time. By combining our expertise in neurotechnology with cutting-edge quantum advancements, we are actively working to shape the future of AI and human-machine interaction.
The Future of Quantum Neural Networks in AI
The future of Quantum Neural Networks holds immense promise, not only in improving the efficiency and capabilities of AI but also in enabling new applications that were once thought impossible. As quantum technology continues to mature, the integration of QNNs into AI systems will drive innovation across industries, from healthcare and finance to transportation and entertainment.
With their ability to process large datasets, optimize complex problems, and enhance pattern recognition, QNNs are set to become a cornerstone of the next generation of AI. As Neuroba continues to lead the way in neurotechnology, we remain committed to exploring the potential of Quantum Neural Networks and their impact on the future of AI.
Conclusion
Quantum Neural Networks are poised to revolutionize the landscape of AI by offering faster, more efficient, and more powerful systems capable of solving complex problems. By leveraging the principles of quantum mechanics and integrating them with neural network architectures, Neuroba is working to shape the future of AI in unprecedented ways. As quantum computing continues to advance, the applications of QNNs will become increasingly vital in driving innovation across diverse fields, transforming how we interact with technology and solve global challenges.
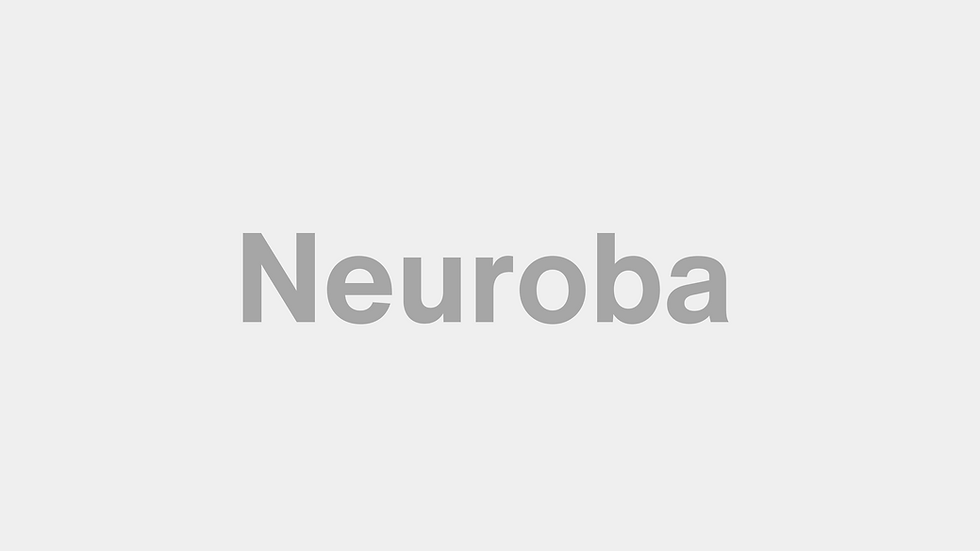
Neuroba: Pioneering neurotechnology to connect human consciousness.